AISym4Med is dedicated to developing a platform that grants healthcare data engineers, practitioners, and researchers access to a reliable dataset system enriched with controlled data synthesis for experimentation and modeling purposes. Emphasizing data privacy and security, the platform integrates cutting-edge anonymization techniques, attribute-based privacy measures, and dependable tracking systems.
In addition to addressing privacy concerns, the platform incorporates measures for controlling data quality, including unbiased data, adherence to ethical norms, context-aware search, and human-centered design for validation purposes. These measures ensure the representativeness of the synthetic data generated. An augmentation module is responsible for exploring and advancing techniques in creating synthetic data dynamically on demand for specific use cases.
Furthermore, the platform leverages federated technologies to replicate unidentifiable data from closed borders, facilitating the indirect assessment of a broader range of databases while upholding privacy, security, and GDPR-compliant guidelines. The proposed framework supports the development of innovative, unbiased AI-based, and distributed tools, technologies, and digital solutions. This aims to benefit researchers, patients, and health service providers while maintaining a high level of data privacy and ethical usage.
AISym4Med contributes to the creation of more robust machine learning (ML) algorithms for real-world readiness, considering optimal computation configurations. Additionally, a machine-learning meta-engine assesses the quality of the generalized model by analyzing its limits and breaking points, enhancing system robustness by providing on-demand real and/or synthetic data. The platform undergoes validation against local, national, and cross-border use cases, catering to the needs of data engineers, ML developers, and aiding clinicians in their operations.
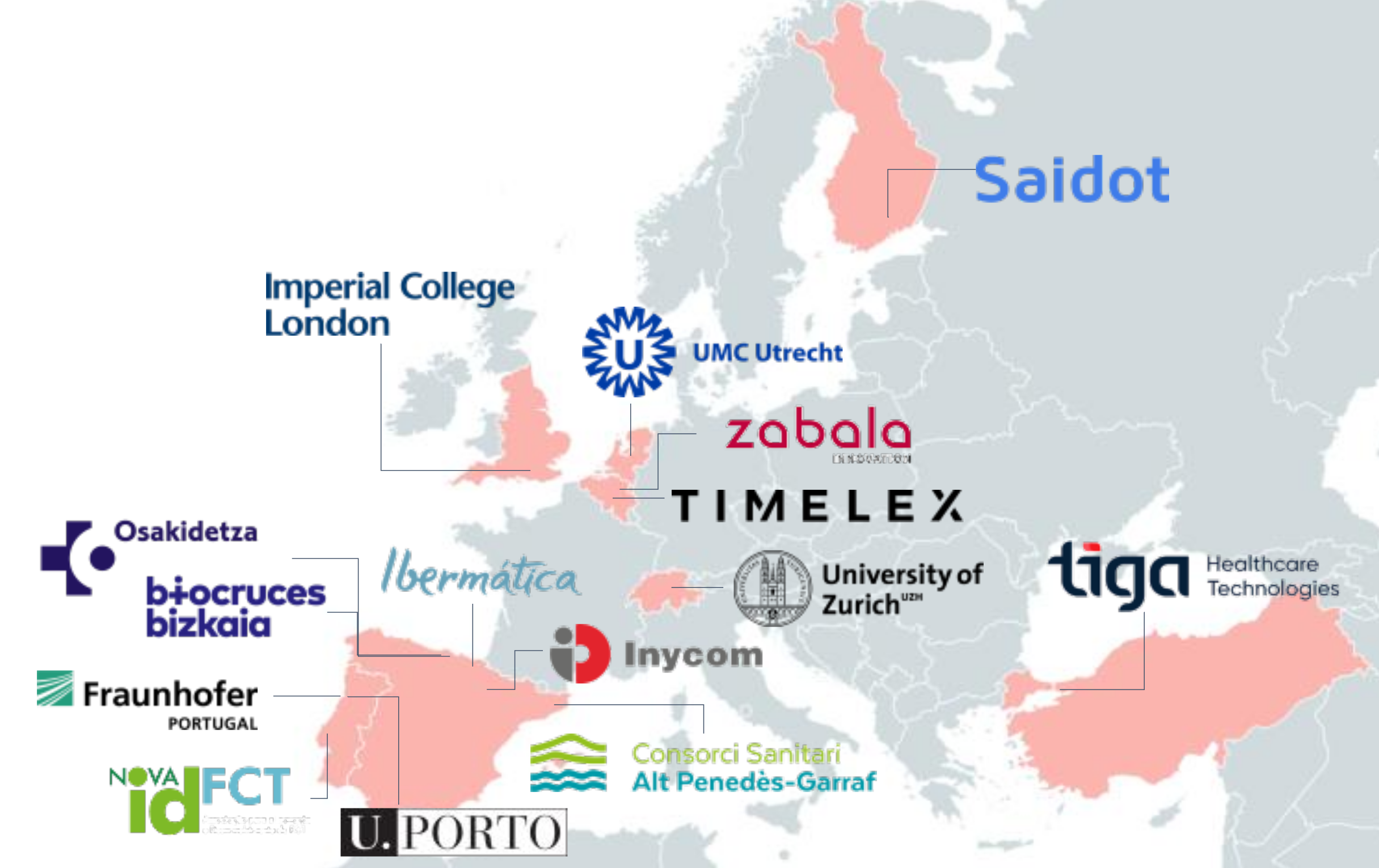
The Communication Systems Group CSG of the Department of Informatics IfI at the University of Zurich UZH leads within Work Package WP1 the Working Group that creates the "Anonymisation Toolkit" to preserve data privacy. Furthermore, in WP1, the CSG leads the "Cybersecurity" working group, which continously evaluates and improves the security of the architecture. Furthermore, the CSG is involved in the Working Groups on "Federated Learning" and "Regulatory Compliance".